I, robot, you, consumer: Measuring artificial intelligence types and their effect on consumers emotions in service
Published in: Journal of Service Research
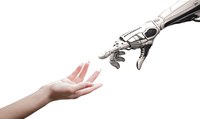
Artificial intelligence (AI) is developed to mimic human intelligence, but what is intelligence? Artificial intelligence is increasingly applied in many sectors and daily activities. Consumers interact with artificial intelligence when they receive product recommendations, run an online search, search for the best path to reach a store, etc.
Psychologists have shown that human intelligence is a multi-faceted concept: people aren’t just more or less intelligent but display different forms of intelligence. Is it the same also for Artificial intelligence? And can tools that evaluate human intelligence inspire new tools to evaluate Artificial Intelligence? This article aims to answer these questions.
The research addresses the following key points:
- applications of AI, with emphasis on the shopping experience
- new tools to evaluate artificial intelligence, identifying 5 AI types
- human emotions to different types of AI intelligence
AI is often developed to simulate human cognitive abilities. Thus, if AI mimics Human Intelligence (HI), a measurement scale for AI should be developed starting from the notions about HI. Yet, the development of tools for measuring or evaluating these different intelligences is still in its infancy.
Likewise, research has yet to determine how people emotionally react when interacting with different AI intelligence types. Thus, the more common human-robot interactions become, the more need to understand (i) what humans perceive about artificial intelligence and (ii) what emotional response such intelligence evokes.
Accordingly, there is a need to investigate the extent to which people evaluate the technology (including AI systems) and how they reply, emphasizing the diverse possible emotional response.
This research provides a combined and more comprehensive overview of the possible new AI types emerging from the contrast with the human one, showing that AI is classifiable against HI, which was not considered in previous AI scales.
Specifically, this research identifies AI types starting from definitions and studies of HI. Unlike other AI classifications, this research evaluates AI against human intelligence. Consequently, its approach expands previous frameworks on artificial intelligence and provides a new and more comprehensive approach to developing AI. For instance, verbal-linguistic intelligence is not the ability to feel empathy or have intuitions: it is the ability to express them efficiently. Thus, it complements extant classifications, separating the ability to feel (Social intelligence), to understand (Logic-Mathematical intelligence), and to express those feelings and intuition verbally (Verbal-Linguistic intelligence). Similarly, this research adds Visual-Spatial intelligence that complements extant classifications, adding the specific ability to understand images and spaces, generate and transform well-structured visual images, and identify movement patterns.
Second, previous studies usually assessed AI intelligence types based on experts’ qualitative opinions. Instead, this research develops a multidimensional measurement scale for AI, similar to the scales of HI, developing a scale to evaluate AI systems’ intelligence types. The results show the extent to which artificial intelligence types are configurable, describable, and measurable, much as is done for human intelligence. Third, this research adds that recent AI-based services can already generate and communicate emotions, and different types of AI lead consumers to different emotions. This evidence sheds light on the emotional transfer occurring during consumer-AI interactions in service, which past studies do not fully cover.
Fourth, this research also highlights that the intelligence types do not symmetrically affect positive and negative emotions: intelligence types inducing the former do not necessarily prevent the latter, and vice-versa. Specifically, Logic-Mathematical and Visual-Spatial increase positive emotions but do not decrease negative emotions. Instead, Processing-Speed reduces positive emotions but does not increase positive emotions. Only Social intelligence affects both positive and negative emotions.
Overall, this research demonstrates that the five AI intelligences differ significantly regarding which emotions they affect and how strongly. The findings answer recent calls to determine how people react and what they feel when interacting with different AI intelligences. In addition, this research extends previous studies on how people are willing to extend real-life psychological dynamics towards artificial entities with evidence from the service context.
In summary, his research draws upon several theories of HI and the Theory of (Human) Emotions and uses retail service as the research context. It extends past studies on AI and emotions by (i) developing a scale to evaluate the dominant intelligences in AI systems, (ii) providing empirical evidence that intelligences for AI can be as diverse as they are for humans, (iii) showing that some AI can display multiple dominant intelligences simultaneously, contrary to humans; and (iv) demonstrating the extent to which consumers show different reactions with different intensities to different AI intelligences, in terms of positive-negative emotions, emotional attachment, satisfaction, and technology continuation intention.
For practitioners, it is important to mature the awareness that different types of AI exist and they solicit different reactions in consumers. Indeed, consumers form different opinions about the type and amount of intelligence of the AIs they interact with. Moreover, interacting with an AI generates emotions in customers, just as when interacting with human personnel. Accordingly, this research recommends that practitioners care about customer-AI interactions no differently than customer-human personnel interactions. Thus, practitioners should consider introducing specific AI types based on consumers’ emotions they want to generate or avoid specific emotional responses. For instance, the research shows that Social intelligence generates emotional attachment to the service provider and does so more than Verbal-Linguistic intelligence. In contrast, Processing-Speed intelligence does not do it at all but helps reduce negative emotions.
Read the full article.
Authors at the Department of Management
DANIELE SCARPI – Associate Professor
Academic disciplines: economics and business management
Teaching areas: Marketing
Research fields: Consumer Behavior
Daniele Scarpi is an Associate Professor at the University of Bologna since 2014. His research is in consumer behavior and was published in leading journals such as J. Service Research, J. Retailing, Tourism Management, Travel Research, Marketing Letters, and many others. The European Commission, The Conversation (UK), and the World Health Organization divulged some of this research.